Organizations need to guard against not only outsider cybercriminals but also rogue insiders. To effectively deal with insider threats, a layered security approach leveraging both preventive and detective security controls should be in place. LOGTITAN focuses on insider threats, such as employees who have already been compromised or malicious employees who have gone corrupt and carry out targeted attacks.
LOGTITAN’s User Behavior Analytics module (UBA) continuously analyzes behavior across multiple users to establish a historical baseline for each user.
LOGTITAN uses machine learning-powered profiling algorithms to build a behavior pattern for each user and entity. LOGTITAN profiles user and entity behavior by looking at 23 different categories including but not limited to authentication, network access, firewalls accept/deny, application activity, port or network scans, denial of service type events, malware or other malicious software activity, etc. This historical information is used to spot any anomalous behavior when there are deviations from the “normal” patterns.
LOGTITAN collects and identifies users’ behavior patterns, organize users into groups, and compare them to the standard ones to identify deviations and violations. The larger the array of data on user behavior is, the more accurately the behavior model will be built, which will, in turn, allow more accurately predict slight deviations from the norm and reveal the suspicious users’ behavior.
LOGTITAN also calculates averages, standard deviations, and other stats dynamically over time, so that they reflect possible shifts in the historical trends.
For example, here’s a possible behavioral rule: Alert when a user deviates from their baseline of normal activity while authenticating to the systems.
This could be translated more precisely as ‘notify, when a user’s last hour logon count is two or more standards of deviation away from their mean or %100 more than the same user’s maximum logon count’.
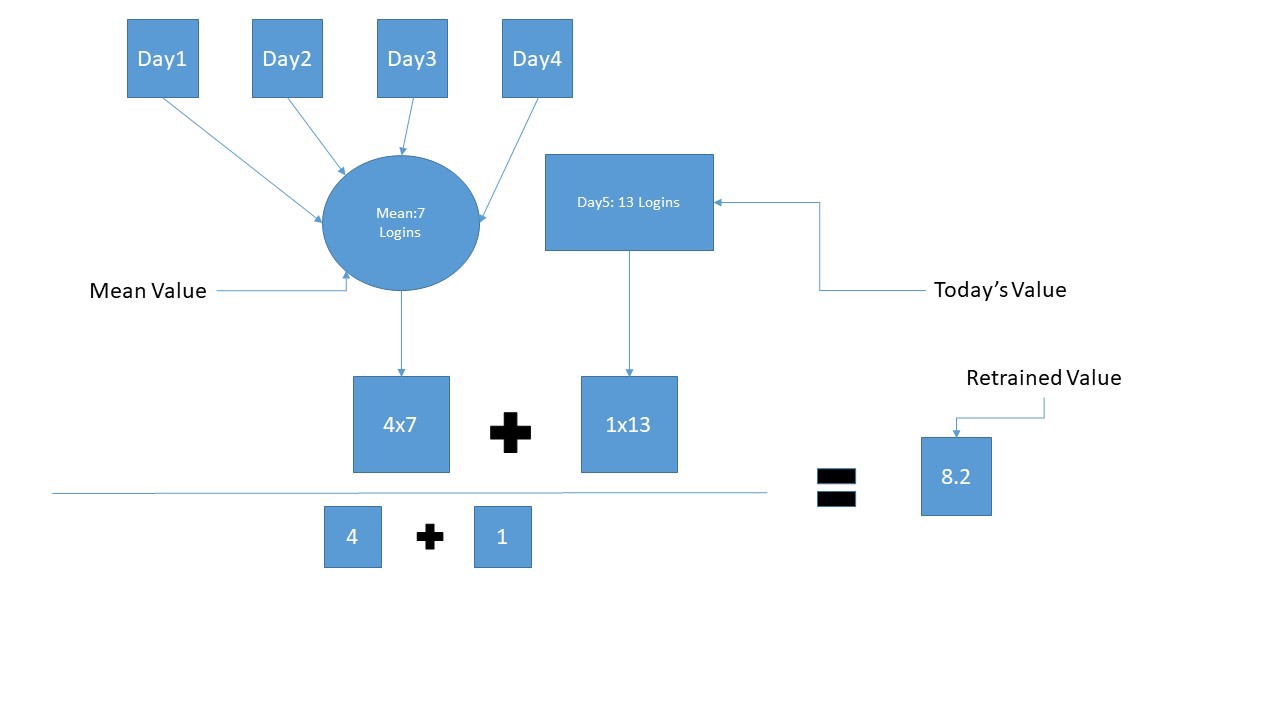
LOGTTIAN effectively addresses insider threats by leveraging machine learning, context enhanced correlation, user and entity behavior detection, and fast security incident investigation capabilities. LOGTITAN can help your organization detect data breaches, privilege user abuse, and sabotage made by insider threats.